SATOMI - Tackling the segmentation and tracking challenges of growing colonies and microbial diversity
Funded by the Helmholtz Imaging Platform
Contact: Hanno Scharr
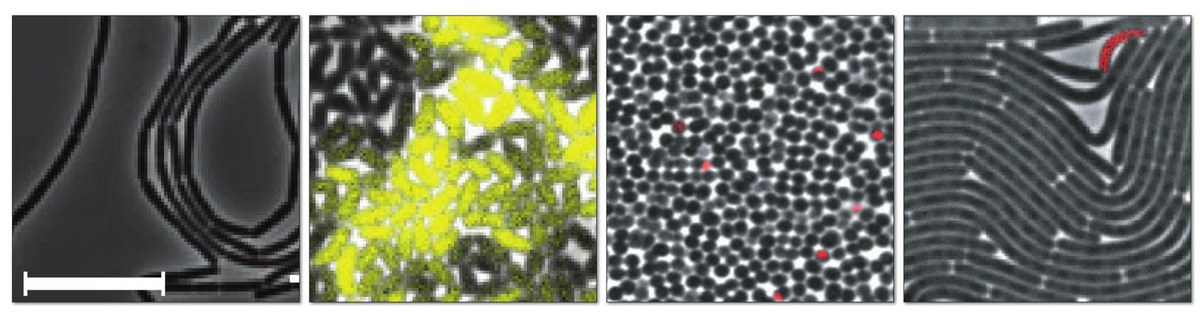
Live-cell imaging is unlocking fascinating insights into living bacteria as they grow and divide in expanding colonies, with morphologies ranging from simple cocci and rod shapes, to multicellular organisms developing into complex filamentous networks. Coupled with highly multiplexed microfluidic lab-on-chip systems, time-lapse microscopy imaging offers several unique possibilities for microbial research: i) to monitor the dynamic growth of bacterial populations non-invasively starting from a single cell on, ii) to capture the evolution of spatio-temporal characteristics of cells and bacterial colonies over long time windows, iii) to derive distributions of ancestral relationships between cells in a populations (lineages), and iv) allow for high-throughput data collection under precise control of the environment. Clearly, the reliable analysis of the collected time-lapse images is essential for extracting quantitative single-cell metrics and turning them into biological discovery. Two basic, tightly linked image analysis tasks to tap insights from the wealth of acquired data are cell segmentation, the spatial identification of individual cells in the images, and cell tracking, the association of these cells and their progeny throughout an image sequence.
The SATOMI platform is designed to expand the range of use cases and to accelerate the time-to-insight in the field of microbial single-cell analysis by:
- Adaptation of state-of-the-art DL-based segmentation methods for bacterial cell segmentation resulting in specialized DL-models for the task of microbial cell segmentation
- Putting probabilistic tracking into production
- Delivering a comprehensive and well-annotated benchmark data set featuring a variety of organisms, and a flexible cell simulator to benefit DL-model generation.
The main goal of the proposed approach is to provide the first benchmark data set and the deep learning-based framework SATOMI for the segmentation and DL-assisted tracking of diverse microbes with different morphologies. Such framework should be able to reach good accuracy already for new use cases, ideally without any additional annotation effort. Nonetheless, the envisioned solution is sufficiently flexible to be later extended by annotated or curated training data. The notorious shortage in manually curated high-quality data is alleviated by a simulator-based approach for near-realistic rendering of cell morphologies and colony growth behavior to yield test image sequences for both, segmentation and tracking. Combined, such a framework is aimed at avoiding the recurring development of highly specialized “one-off” tools for new use cases from different domains such as biotechnology, environment, and health.
Cooperation partners:
Katharina Nöh, Forschungszentrum Jülich, IBG‐1: Biotechnology
Dietrich Kohlheyer, Forschungszentrum Jülich, IBG‐1: Biotechnology
Ralf Mikut, Karlsruhe Institute of Technology (KIT), Institute for Automation and Applied Informatics (IAI)